Our Investment in Tecton
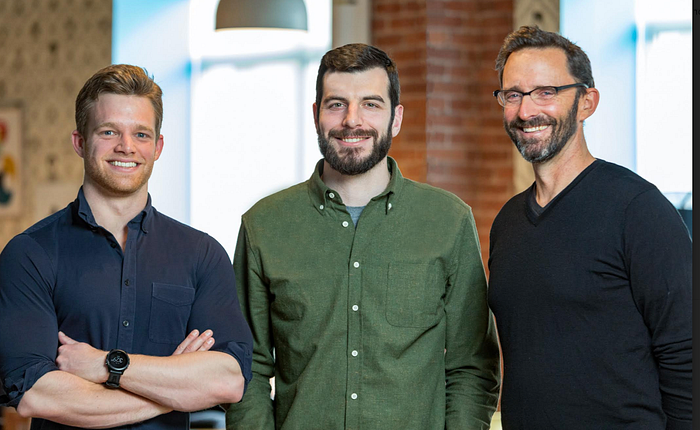
Much of the past two decades of innovation and evolution in data infrastructure have been born out of the largest tech companies. Google and Yahoo were credited for the Hadoop platforms — Facebook built Cassandra and Presto to store and query data at large volumes — Kafka was created inside LinkedIn — and Uber quickly scaled and operationalized machine learning across the company.
For many enterprises, running machine learning in production has been out of the realm of possibility. Talent is scarce, the state-of-the-art is evolving rapidly, and there is a lack of infrastructure readily available to operationalize models. While some tech companies have been running machine learning in production for years, there exists a disconnect between the select few that wield such capabilities and much of the rest of the Global 2000. Some internal ML platforms at these tech companies have become well known, such as Google’s TFX, Facebook’s FBLearner, and Uber’s Michelangelo. What many of these companies learned through their own experiences of deploying machine learning is that much of the complexity resides not in the selection and training of models, but rather in managing the data-focused workflows (feature engineering, serving, monitoring, etc.) not currently served by available tools.

We at Lux have a history of investing in companies leveraging machine learning. In addition, our experience and the lessons we’ve learned extend beyond our own portfolio to the Global 2000 enterprises that our portfolio sells into. Any time there are many disparate companies building internal bespoke solutions, we have to ask — can this be done better? More specifically, to identify the areas of investment opportunity, we ask ourselves a very sophisticated two-word question: “what sucks?”. Tooling to operationalize models is wholly inadequate. The story we often hear is that data scientists build promising offline models with Jupyter notebooks, but can take many months to get models “operationalized” for production. Teams will attempt to cobble together a number of open source projects and Python scripts; many will resort to using platforms provided by cloud vendors. What we noticed is missing from the landscape today (and what sucks) are tools at the data and feature layer. A whole ecosystem of companies have been built around supplying products to devops but the tooling for data science, data engineering, and machine learning are still incredibly primitive.
Tecton was founded by Mike Del Balso, Jeremy Hermann, and Kevin Stumpf, who met at Uber and were responsible for building Michelangelo, Uber’s large scale internal machine learning platform. Michelangelo supported 100+ use cases and over 10,000 models in production, applying machine learning to problems such as improving user experience, ETA prediction, and fraud detection. At Uber, the team noticed engineers spent a majority of their time “selecting and transforming features at training time and then building the pipelines to deliver those features to production models”, which is a problem we have heard repeatedly echoed by other companies across industries. Tecton is focused on solving these issues and beyond by building an enterprise-ready data platform to help teams operationalize machine learning and enable data science and engineering to collaborate efficiently.
Managing data and performing operations such as feature discovery, selection, and transformations are typically considered some of the most daunting aspects of an ML workflow. Michelangelo had a concept of a “feature store” to ease these problems by creating a central shared catalog of production-ready predictive signals available for teams to immediately use in their own models. Solving the common issue of “development in silos”, this platform brought a layer of standardization, governance, and collaboration to workflows that were previously disconnected. Similarly, Tecton wants to bring best practices to the data workflows behind development and operation of production ML systems. The platform will provide any enterprise — no matter how large or small — with the ability to supercharge their machine learning efforts, empowering them with similar infrastructure and capabilities otherwise only available to large tech companies
Tecton’s mission is to make world-class machine learning accessible to every company. We are proud to join in their $25M seed + series A raise and are thrilled to partner with Mike, Jeremy, Kevin, and the rest of the team on this journey.
Follow the team on twitter at @TectonAI.