National security and the decline of the VC exit
Exits are the keystone of a robust venture economy. Ambitious entrepreneurs, helpful accelerators and fast-funding venture capitalists are all necessary inputs of course, but what attracts all this talent and capital in the first place is the opportunity to build something incredible and actually make money (and preferably a lot of it) while doing so. Startups are a pipeline from conception and product-market fit to scaling and exit, and it’s that final stage which creates the pressurized vacuum pulling each company from the beginning of the pipeline to the end.
Many cities — and even nations and entire continents — get this mind-bogglingly backwards. Governments often invest in incubators or community workspaces to network founders and investors together. That’s well-intentioned, but entirely the wrong focus. What can instantly catalyze this activity is the alignment provided by massive potential future returns at the lowest possible risk.
The United States and China have been the strongest global markets for startup innovation over the past two decades. Both have offered extraordinary returns on entrepreneurial effort and ambitious risk capital: the two countries are the only ones in the world which have ultimately crowned venture-backed tech giants worth hundreds of billions of dollars. As one generation of entrepreneurs and executives build their wealth, they have strong incentives to invest in the next generation of founders looking for their own returns.
In a peculiar harmony though, both countries are implementing policies to shrink potential exits, diminishing the rewards of entrepreneurship and ultimately thinning the potential innovation their dynamic markets create.
China’s policy of “common prosperity” has been covered extensively, and if you haven’t followed all the ups and downs, Ryan Haas at the Brookings Institution published a succinct overview from last September. In short, there is on-going action from the central government to redistribute the returns from the country’s leading tech companies like Alibaba and Tencent to the broader population, ostensibly to reduce inequality but also beneficially squelching the growing power of tech companies in relation to the Communist Party of China.
China is not alone in harming its local startup ecosystem though. Under the rubric of national security, the United States is pursuing policies that will diminish exit values and will damage the long-term competitiveness of its own technology industry.
The damage started with a 2018 law called FIRRMA, which expanded and strengthened the U.S. government’s oversight of foreign investors buying equity in American companies. The law’s intention is to prevent foreign adversaries (namely China) from purchasing American companies (in whole or in part) and acquiring their technical know-how in critical technologies, such as semiconductors and quantum computing. Given the great power competition between the U.S. and China, it’s understandable that America wants to prevent its best innovations from falling into the hands of a hostile power.
As always with such laws though, its limits are blurry. SoftBank and its Vision Fund, which for a period was the most prodigious investor in global startups, was tangled up in the regulations, since SoftBank is a Japanese-headquartered conglomerate and the Vision Fund also drew heavily from Middle Eastern sovereign wealth. Japan may well be a mutual defense treaty partner, but from the U.S. regulatory perspective, any foreign country from Canada to North Korea could potentially be a threat to American industry.
FIRRMA’s direct effects then are to decrease the supply of capital into America’s critical technology industries and also, by definition, reduce their exit values by partially restricting the number of bidders for a company’s equity. In other words, the law limits the potential upside of any startup venture in the industries with the most technical risk, precisely the companies where venture dollars are often the hardest to raise.
Right now on Capitol Hill, Congress is debating an even tougher piece of legislation that would go beyond restricting foreign investment into the United States, but also outbound engagement. From the Wall Street Journal article:
The revised screening measure would enable the federal government to restrict certain future transactions in any “country of concern,” defined as “foreign adversary” countries including China, according to the new text. The provisions would apply to greenfield investments, such as the construction of new plants, to deals such as joint ventures that involve the transfer of knowledge or intellectual property and to capital contributions including venture capital and private equity transactions, the text says.
What are some of the sectors covered right now? The Journal continues:
Those sectors and technologies include semiconductors, large-capacity batteries, pharmaceuticals, rare-earth elements, biotechnology, artificial intelligence, quantum computing, hypersonics, financial technologies and autonomous systems such as robots and undersea drones.
So essentially everything but 15-minute delivery apps (or maybe that falls under hypersonic?)
China, of course, is a serious threat to U.S. global hegemony. Its economy remains robust in spite of massive Covid-19 lockdowns, its consumer market has expansive room to grow, and it has an incredibly sophisticated and competitive industrial policy to build capabilities in the most important sectors of future technology. America needs to compete, and compete very effectively, if it wants to hold on to its leadership positions.
But consider what the effects of the law would have on American startups. More sources of venture capital would be restricted. Potential product sales could be blocked, diminishing revenue growth. Exits are further complicated, particularly given that the law could potentially cover any company that is partially owned by Chinese nationals or sources. At every stage of the venture pipeline, growth and value will be clipped.
That diminishment of potential will have ramifications for both VCs and founders. As more and more semiconductor and quantum companies struggle against American bureaucracy to grow and exit, VCs — already stereotypically weary of investing in these sorts of capital intensive verticals — would be wise to consider whether more unregulated innovation hotspots like crypto are a better bet. Founders similarly will look at the regulatory crackdown and maneuver where they can build freely. Why deal with the uncertainty and red tape of the Treasury Department, particularly when building a startup takes a decade or more?
Congress is facing incredible pressure from a range of business groups to narrow the scope of the bill, whether it be the countries or industries or the types of transactions covered. Yet, the general tenor of Washington these days is clear: more restrictions and more control.
That’s not surprising given China’s looming and lethal threat, but frankly, it’s also decades too late. At this point, in 2022, the idea that China’s rise can be hindered by export controls and government reviews is highly circumspect. Such restrictions would have been incredibly effective in the 1990s and 2000s when China’s nascent internet market and tech industry were just getting off the ground. That window of opportunity has long since closed. Now, the only question should be pursuing policies that strengthen America’s startup ecosystem relative to all other countries, and ensuring that we have the most dynamic, robust, and lucrative innovation economy in the world. This latest bill in Congress is a step in the wrong direction.
Lazy tech analogies
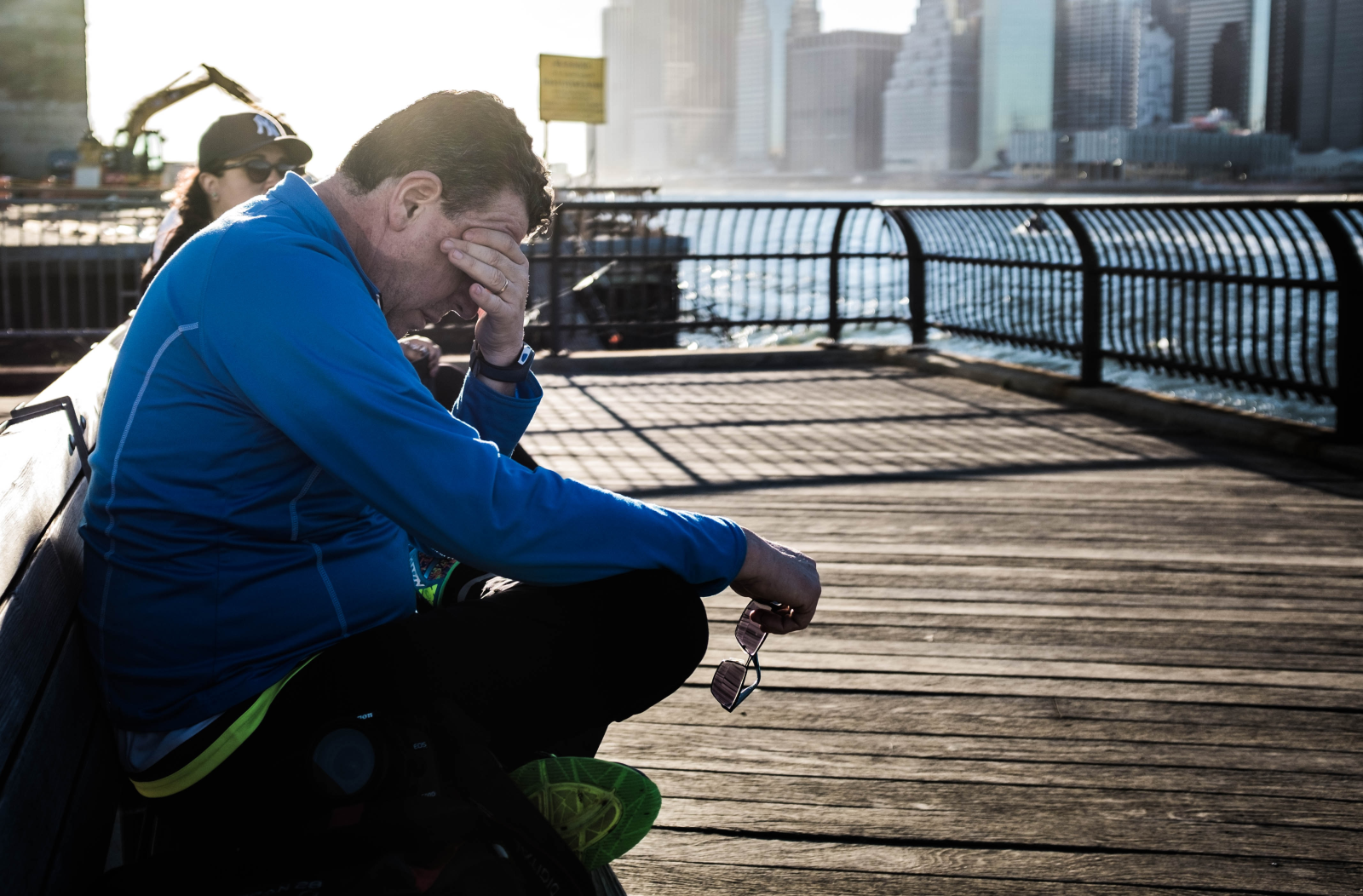
I want to briefly follow up from last week’s “Securities” newsletter on “Marginal Stupidity” (which we also discussed in a new podcast episode). The world is incredibly complicated, and humans necessarily use heuristics and analogies to process that complexity into simpler forms. These mental shortcuts are never perfect, but they should broadly summarize the complexity they represent while affording their user a sense of their limitations.
In that vein, I want to call attention to two lazy tech analogies that I’ve seen lately as examples of the kind of impoverished analogical thinking that the industry needs to actively avoid.
The first was the wild story about Blake Lemoine, an engineer at Google, who claimed that the AI system he was conversing with was sentient. This story went supernova viral, and there have been some great pieces explaining why artificial general intelligence (AGI) is not present in this example.
At the heart of the misunderstanding is the lazy analogy of “sentience.” Philosophers can rightfully debate that topic forever, but an advanced AI language model that is very good at stringing together reasonable responses to conversational input is absolutely not sentience. Borrowing from software programming theory, what we have here is what might be dubbed the logical mistake of duck typing (“If it walks like a duck and it quacks like a duck, then it must be a duck”). Appearing sentient, and actually being sentient, are two different things, regardless of what the Turing test might argue.
Second, and this comes via Shaq Vayda, is the continuing usage of the word “cloud” to describe a range of startups in wetlab biotech. The idea of “wetlab clouds” is to allow software engineers to programmatically operate a bio lab, using API calls to string experimental steps together like pipetting, northern blotting a sample, or conducing PCR. Writing code would be easier, faster and more repeatable, drastically increasing the efficiency of the biolab.
The wetlab cloud is a nice metaphor, but one that occludes too many complexities. Biological scientists do an incredible amount of work to avoid cross-contamination, to calibrate their instruments, and to adjust their experiments on the fly to account for the regular variabilities in bio research. Those tacit activities are not easily reproducible, and certainly not in the effortless sense of a “cloud.” Wetlab automation is an important category of investment, but analogizing it to software is wrong.
These two examples are just some of the litany of bad analogies roaming out there, particularly in crypto. These are not useful mental shortcuts, but rather dangerous metaphors that can actively blind us to some of the most challenging facets of these complicated industries. We all need to be vigilant of these elisions and dive deeper when we encounter them.
Lux Recommends
- Shaq recommends Emily Mullin’s discussion in Wired on new research focused on epigenetic editing. CRISPR can allows scientists to precisely snip strands of DNA, but epigenetic editing allows them to leave the DNA in place while modulating its expression, potentially opening up a new domain of therapeutics.
- Grace Isford calls attention to Ethereum co-founder Vitalik Buterin’s new piece on the non-financial usages of blockchain. Meanwhile, I’d bring attention to a newish working paper published by him along with E. Glen Weyl of Microsoft Research and Puja Ohlhaver of Flashbots on a blueprint for using Web3 to decentralize society (shortened to “DeSoc”).
- Shaq also points out this Nature interview by Chris Woolston on the decade-long rise of the research software engineer, or coders who build out the tooling and infrastructure for cutting-edge laboratory work.
- In a useful corrective, Lada Nuzhna, writes on the over-argued potential for deep learning to change biotech and biochem. “I have been periodically noticing an impression that for ages biology and chemistry have been done with rocks and sticks in caves until deep learning was brought to wet labs by computer scientists, akin to Prometheus bringing fire to mankind.”
- Finally on that old pulp medium of the book, Alexander Larman has a scorching take on literary festivals in “Sheer hell in a tent,” the FT explores bibliographic influencers on “BookTok”, and Bill Morris argues that spending tens of thousands of dollars on bookshelf consultants is not the madness it clearly is.
That’s it, folks. Have questions, comments, or ideas? This newsletter is sent from my email, so you can just click reply.